AI expands reach of SDB
Satellite-derived bathymetry (SDB) technology has been improved and applied more substantially in the past three years than in the previous two decades, primarily due to increasingly accessible machine learning algorithms, scalable computing power, a multitude of satellites orbiting the Earth and a new source of validation data. Today, SDB is applicable in water conditions and geographic areas once considered entirely unsuitable for the technology.
Credit for these recent advancements must also be attributed to funding by the U.S. government. In 2018, the National Science Foundation (NSF) awarded a Small Business Innovation Research (SBIR) grant to TCarta Marine of Denver, Colorado, to initiate Project Trident. Its goal was to leverage machine learning to expand SDB beyond clear, calm waters into more dynamic, turbid coastal environments. [Project Trident was described in the June 2020 issue of Sea Technology.]
As several key components of the research came to fruition, TCarta set its sights on Alaska. SDB had rarely been applied successfully in this state due to a combination of factors, of which water clarity is just one. The National Oceanic and Atmospheric Administration (NOAA) awarded SBIR grants to TCarta in 2020 and 2021 to adapt and modernize SDB techniques and incorporate new sensor capabilities for Alaska and the Arctic region.
This article describes the latest progress from Project Trident and the current state of play for SDB in northern latitudes.
What is SDB?
SDB technology extracts water depth measurements from satellite imagery at lower cost and with fewer restrictions than ship-borne sonar or airborne LiDAR. SDB algorithms are applied to what is called ‘multispectral’ optical satellite imagery. Multispectral means the onboard sensor measures natural energy reflectance from features on the Earth’s surface in many wavelengths, usually visible Red, Green, and Blue, as well as near infrared.
SDB is now a commercial technology, and TCarta routinely generates SDB products with bathymetric point values spaced less than a meter apart on the seafloor to depths of 20 meters, depending on water clarity and turbidity. In extremely clear water, measurements down to 30 meters are reliably produced. The accuracy of these data sets is typically 10-20% of water depth.
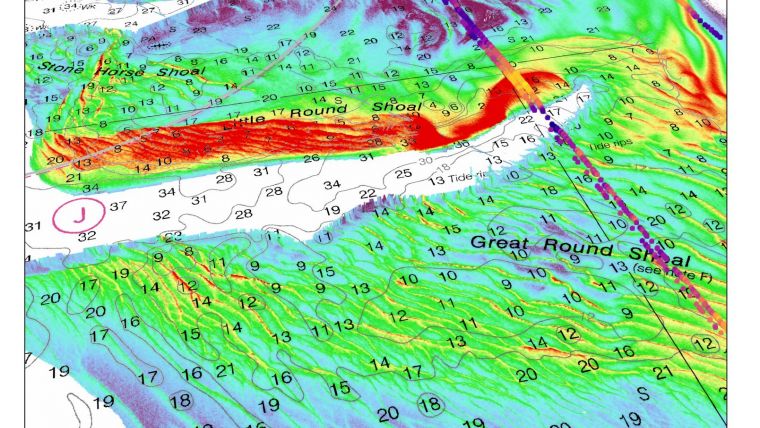
Enhancing SDB with ML and ICESat-2
SDB processing of multispectral imagery has primarily involved application of two algorithms – Band Ratio and Radiative Transfer. Both are mathematical calculations that rely on visible light passing through the water column and reflecting off the seafloor. The drawback, of course, has always been the fact that light doesn’t penetrate murky, sediment-loaded waters in many dynamic coastal environments.
With the NSF-funded Project Trident, TCarta saw supervised machine learning (ML) as a solution to this problem on many fronts. Explained simply, supervised ML uses verified training data to teach the computer to recognize similar features or patterns in other comparable data sets. It is important to note that the current ML renaissance has been made possible by widespread and affordable availability of the massive computing power required to run the iterative ML algorithms in reasonable periods of time.
The underlying premise of Project Trident was that coastal zones known for their cloudy, turbid waters traditionally deemed unsuitable for SDB likely experience at least a few days of water clarity each year. It was simply a matter of collecting satellite imagery, or finding imagery already acquired, on those rare days.
This is where imagery from the European Sentinel-2 satellite came into play. This satellite collects imagery constantly, building an archive of hundreds of images for each spot on the Earth’s surface since its 2015 launch. More importantly, the data is available globally for free.
TCarta technicians created ML-based software to automatically search the Sentinel-2 archive and select those coastal images with water quality appropriate for SDB. The same filtering could be accomplished with the naked eye but would literally have taken years compared to what ML can do in minutes in the cloud or on a computer equipped with an AI-capable graphics processing unit (GPU).
The automated ML image filtering was a success and, in many cases, returned not one, but dozens or even hundreds of suitably clear images. With the capability to analyze the full archive of available imagery, TCarta’s technicians found a substantial advantage when the ML method was scaled to use not just one good image, but to identify suitable individual pixels within multiple images that could be used for SDB processing. These filtering techniques are referred to as pixel-wise and image-wise.
TCarta remote sensing scientists also worked on enhancing the SDB process itself. The team customized a common ML-based decision tree algorithm called Random Forest and adapted it for extraction of water depths from the multispectral images. Random Forest thus became an SDB calculation complementary to Band Ratio and Radiative Transfer.
The Colorado firm then upended the entire SDB workflow. Rather than apply one algorithm to a single satellite image, they created composite images of the best pixels. Then they leveraged the power of cloud computing to process multiple SDB algorithms on every suitable pixel selected by the filtering. This meant dozens of water depth calculations were typically made for each point on the seafloor that was visible in the imagery.
Highly accurate bathymetric values were then determined by combining the results of the multiple calculations. While initially developed and deployed on Sentinel 2 imagery, these same techniques and methods can be applied to any optical imaging satellites
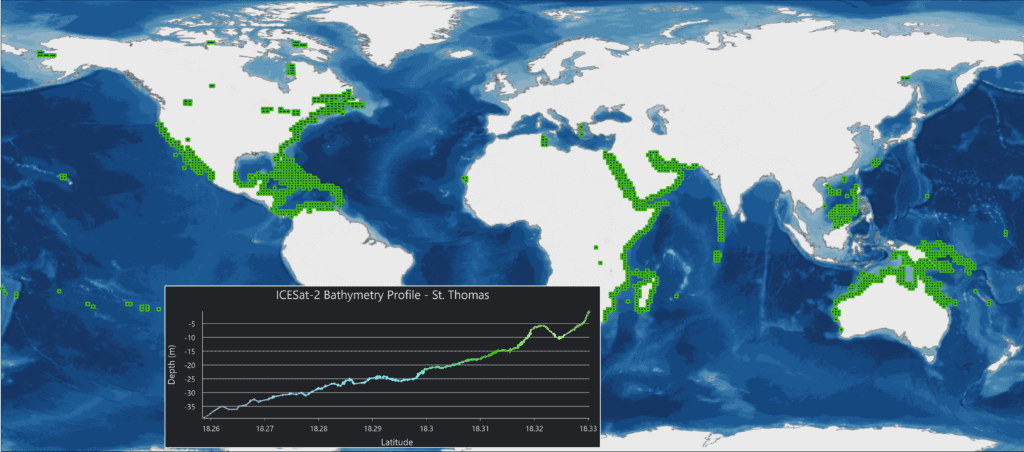
Enter ICESat-2
An addition to the SDB workflow came with the 2018 launch of the NASA ICESat-2 (Ice Cloud & land Elevation) satellite carrying a laser altimeter, or LiDAR, designed for measuring the thickness of sea ice, glaciers, and tree canopies. The ICESat-2 data proved extremely adept at determining elevation points for the seafloor at 0.7-meter intervals with centimeter-level accuracy to depths of 30 meters. Although not applicable for broad-area mapping due to the large gaps between measurement lines, this free data was ideal as a ground truth data set for Project Trident and scalable and global extraction and application of these data may be the single most important SDB technology breakthrough of the research.
TCarta integrated the satellite LiDAR data into its SDB workflow in two important ways. First, ICESat-2 bathymetric measurements were employed as training data sets for the ML-based Random Forest algorithm. Then after the elevation extraction processing was complete, the team used ICESat-2 points to validate the accuracy of overall SDB calculations, reducing uncertainty in derived water depth values.
As a result of Project Trident, TCarta has generated bathymetric maps for coastlines where water conditions had traditionally thwarted SDB application. These areas include the Korean Peninsula, Greenland, Arctic Canada, and New England in the U.S. The depth and accuracy of these locations can now equal those that SDB has previously delivered in clear, calm water.
The research culminated in the launch of a new Global Satellite Derived Bathymetry (G-SDB) product extracted from Sentinel-2 and ICESat-2 data. G-SDB data set contain bathymetric measurements from 0-20 meters generally and up to depths of 30 meters, depending on water clarity, at 10-meter resolution. The depth values for every 10-meter pixel are the combined result of numerous measurements, resulting in accuracy within 10% of depth or less. G-SDB will be available globally for all oceans and seas, as well as large freshwater lakes where water conditions permit.
Applying the results of Project Trident, the Office of Coast Survey at NOAA contracted TCarta to deploy the technology to produce 2m resolution SDB using WorldView imagery for two historically difficult areas of Western Lake Michigan and around Cape Cod and Nantucket Sound, where natural forces alter the underwater terrain faster than traditional bathymetric surveys can be completed SDB is being considered as a fast and inexpensive alternative for such coastal zones.
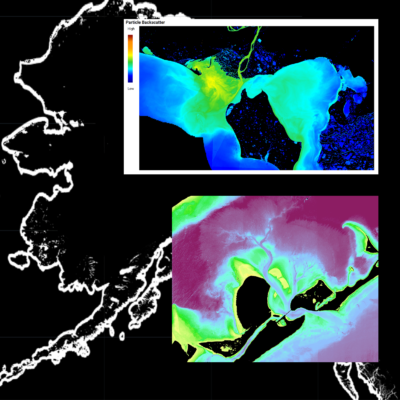
Fine Tuning SDB for Alaska
Mapping Alaska’s coastal waters became a priority for NOAA in December 2019 with the issuance of a Presidential Memorandum which resulted in the development of the Alaska Coastal Mapping Strategy and cites SDB as a key technology for surveying the 32,000 miles of Alaskan shoreline.
In 2020, NOAA awarded an SBIR grant to TCarta to research and deploy multiple SDB techniques and approaches for Alaska. The Arctic region was considered almost impossible for SDB implementation due to a multitude of factors. Coastal waters there are extremely dynamic, carrying excessive sediment loads, not to mention sea ice, nearly year-round. Additionally, six months of darkness and frequent cloud cover thwart image collection by optical satellites.
The goal of the NOAA Phase II grant is to determine how best to deploy SDB techniques in Alaska and if the ML algorithms and pixel-wise filtering techniques can be refined for use with higher-resolution commercial imaging satellites in Arctic waters. Even more ambitious, TCarta scientists hope to create composite bathymetric surfaces composed of the best combined pixels from multiple different satellite sensors.
In the first phase of the research, the project is focused on determining when is the optimal time to capture imagery for the most accurate SDB surfaces and comparison of accuracy across multiple optical satellite sensors.
Timing of imagery collection with the tidal phases is paramount in Alaska due to the significant range and because the best images for use in SDB are captured in sync with incoming tides. This is preferable because the maximum tidal zone is exposed and the water is shallow farther out, allowing SDB to measure depths at a greater distance from the shore. The incoming tide typically has better clarity because it brings in clearer ocean water and typically carries less sediment runoff from the land.
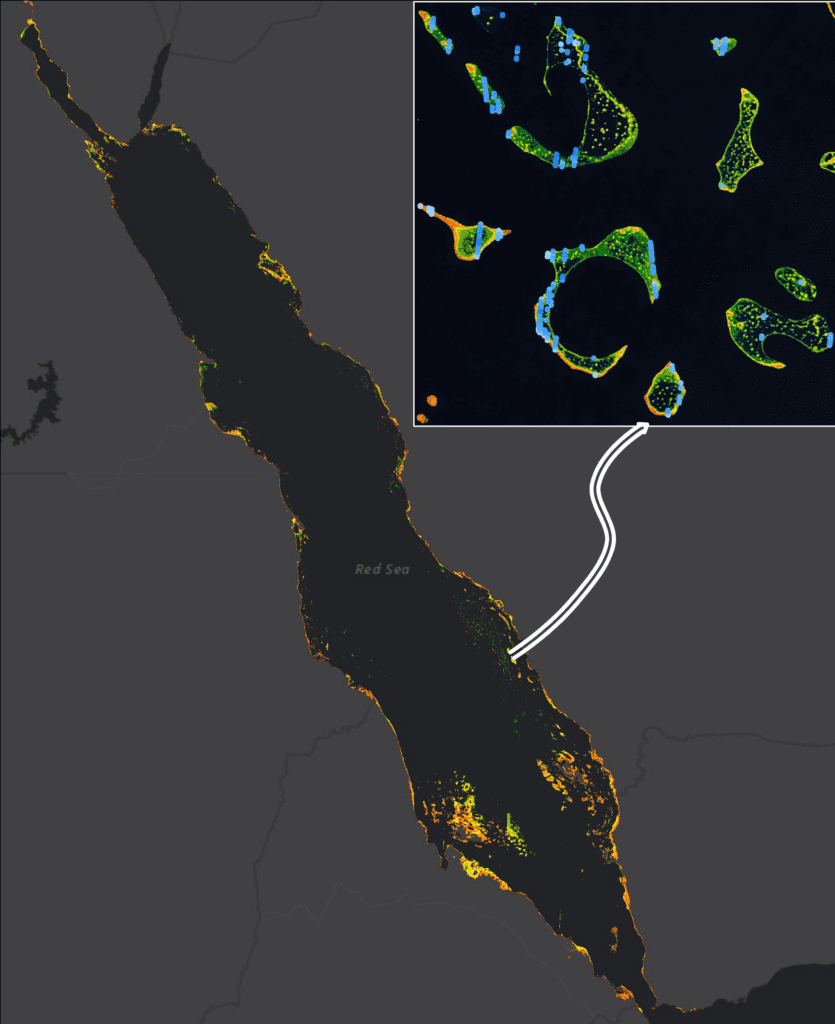
The second phase of the Alaska research is to determine when – or if – optical imagery suitable for SDB processing has been or will be acquired for coastal Alaska. Between the tidal and environmental conditions, TCarta knows the likely collection window is so small that it will not find a treasure trove of hundreds, or even dozens, of appropriate images in existing archives.
To increase the chances of obtaining the necessary high-quality imagery, TCarta is once again applying its ML-based search capabilities to review images and individual pixels acquired by high-resolution optical satellite programs: MAXAR’s WorldView and Planet’s SkySat, most notably. They are also beginning to incorporate hyperspectral imagery – covering broader wavelengths – from Satellogic, a new provider in commercial remote sensing.
The remote sensing scientists realize they may end up with a combination of SDB-worthy images and pixels from multiple sensors to process together to calculate water depth in small coastal areas.
With this in mind, TCarta had developed a process to assimilate radiometric response to compensate for sensor differences among the various satellite systems. This will enable the ML filtering algorithms to make apples-to-apples comparisons between Planet, Satellogic and WorldView pixels, for example. Assimilation will also be required when SDB processing is applied to stacks of pixels from multiple different satellites.
TCarta is confident that it will make significant headway in determining which satellite sensors, or combinations thereof, have the greatest potential for capturing SDB-quality data. Moreover, the researchers also expect to have begun piecing together a predictive schedule of when the image acquisitions should occur for specific parts of the Alaskan coast. Optimism is tempered by the possibility that suitable images will never be acquired for some areas due to extreme conditions.
Where applicable images – or pixels – are acquired, however, TCarta plans to begin processing them in the refined Project Trident SDB workflow by summer 2022. Again, optimism is modest with expectations suggesting that SDB, if it is successful at all, may produce results in depths of only 8-12 meters and limited to 3-5 meters in some locations.
There will remain areas where clear water conditions never exist. But these data and information results will have a high degree of confidence and will represent an improvement over what is available now for many coastal zones.
A secondary, yet important outcome of the Alaska research is that by using information from multiple satellites, TCarta will be able to predict when and where coastal water clarity is best in the state on a daily basis. The Colorado firm has plans for a web portal for accessing these insights in support of NOAA’s hydrographic surveying operations so it can better plan where to deploy LiDAR mapping aircraft in the region. LiDAR, like SDB, depends on clear water for highly accurate subsurface data collection.
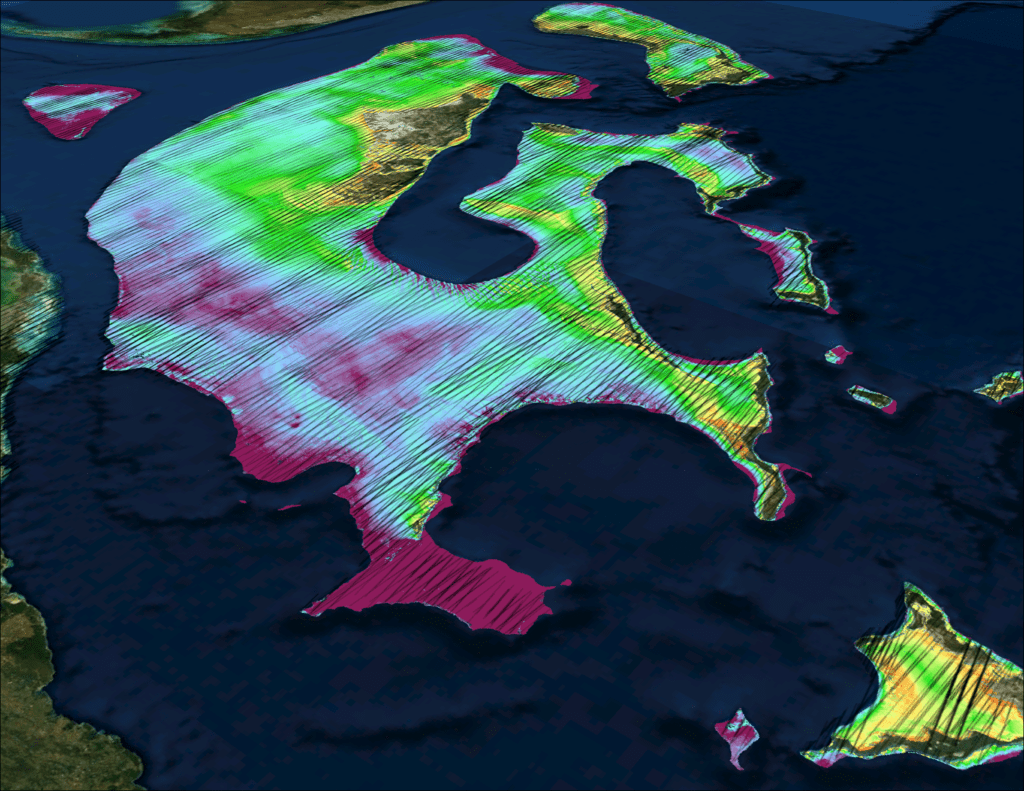
There is no one best solution for surveying coastal water, particularly remote and dynamic areas like Alaska but by combining the recent advancements in SDB technology with the new and next generation space-based sensors along with machine learning and cloud computation, there is great potential for SDB to address in part the challenge of surveying coastal waters in Arctic conditions.
Through both the broad and automated approach to global SDB that TCarta developed under Project Trident and the precision collection and combination of sensors for Alaskan waters, TCarta has developed and deploying a multitude of next-generation approaches to Satellite Derived Bathymetry to address the global need for coastal bathymetry.
View this article here: https://lsc-pagepro.mydigitalpublication.com/publication/?m=60787&i=710019&p=8&ver=html5